In the contemporary manufacturing environment, companies struggle for efficiency and innovation to remain competitive. Digital twins technology is a virtual representation of physical assets and processes and is considered a new trend that is shaping the manufacturing sector through the application of computational and cognitive technology. Digital twins often receive diverse data sources which makes it possible for real-time monitoring, within-the-environment simulation, and optimization, thereby helping manufacturers run their operations smoothly. As such, they provide in-depth insights and better informed decisions, revolutionizing classical manufacturers’ design ideas, production, and optimization. The development of digital twins represents a tremendous milestone allowing manufacturing processes to take on new forms that are, precise, economical, and well-thought-out. This article explores the power of digital twins in modern manufacturing showcasing their essential role in decision making, cost reduction, and enhancing efficiency.
What are Digital Twins?
Basically, digital twins are virtual representations that precisely replicate the attributes and behavior of the physical objects or systems. These are built by combining data from diverse sources including Internet of Things (IoT) sensors, machines, and systems. This information is fed into models to establish a digital replicate that can be observed and run in real-time. Digital twins are not just static models, but dynamic and constantly evolving. As such, when the real-world data from an actual asset or process is consistently inputted into the digital model, it is constantly upgraded and refined to reflect the latest behavior and dynamics. This enables manufacturers to monitor and analyze data through a digital setting, which allows them to see the existing problems, run scenarios, and optimize the processes without distracting or halting production.
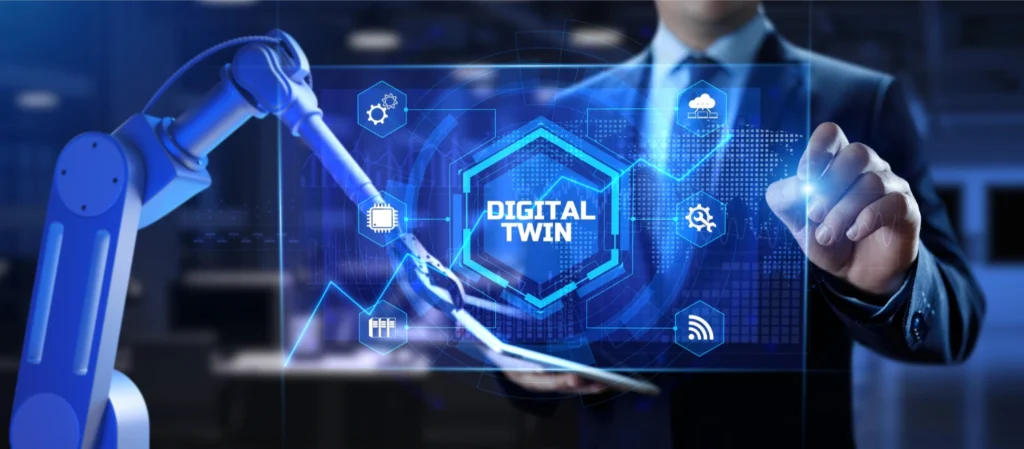
The key components of a digital twin include:
- Physical asset or process: This is the physical entity being virtualized or the prototype in the real world.
- Data acquisition: Sensors, IoT machines, and systems record data about the physical Asset or the process itself.
- Data integration and processing: The gathered data is merged, cleaned, and transformed into usable digital representation.
- Virtual model: The processed data is used to build a virtual representation of the physical device or process.
- Simulation and analysis: With the aid of digital twins, the simulated scenarios can be tested to gather information, perform data analysis, and improve the processes.
- Feedback loop: The digital twin model can be utilized to obtain useful information and resolve bottlenecks, in the process leading to a cycle of continuous improvement.
History of Digital Twins
Digital Twins as a concept can be traced back to the 1990s when David Gelernter came up with this idea in his book titled “Mirror World.” According to him, these would be virtual, large-scale physical environments that could be manipulated and handled by judging reality, using computers. In 2002, Dr. Michael Grieves started working on the practical applications of digital twins in the manufacturing industry by modeling a lifecycle management model around this concept. However, it is worth noting that the term “digital twin” as such was introduced in 2010 in the NASA report by John Vickers. From that time onward, digital twin technology has been used by numerous industries as a means of interacting with actual objects or environments digitally.
Differences between Simulation and Digital Twins
A digital twin differs from a simulation in that it has continuous real-time data integration, bidirectional data flow as well as lifecycle representation, and is linked with data from multiple sources. On the contrary, a simulator is based on constant inputs, whereas a digital twin constantly updates the sensors with real-time information thus enabling a two-way exchange of information between the virtual model and the real asset. This enables the creation of the digital twin, which sends the signal of the current system status and its working pattern. Digital twins attempt to cover a complete life cycle, which begins with design and passes through operations and decommissioning, and integrate info from different sources such as design specifications, sensor data, history, and environmental conditions. Conversely, simulations are usually concentrated on specific stages or parts and are based on a limited set of input variables.
Types of Digital Twins
Digital twin applications in manufacturing can be categorized into different types based on their scope and level of analysis:
• Component Twins: These are virtual copies of each individual component, which gives access to details such as real-time and historical information across the production line.
• Asset Twins: These are product and assets’ exact replicas based on multiple component twin models, and therefore it becomes feasible to optimize production and maintenance.
• System Twins: These twins model interactions among different assets within a manufacturing system to achieve high efficiency and productivity.
• Process Twins: These are high-level representations of an entire manufacturing line or factory, inclusive of all operations and assets, enabling process monitoring and improvements.
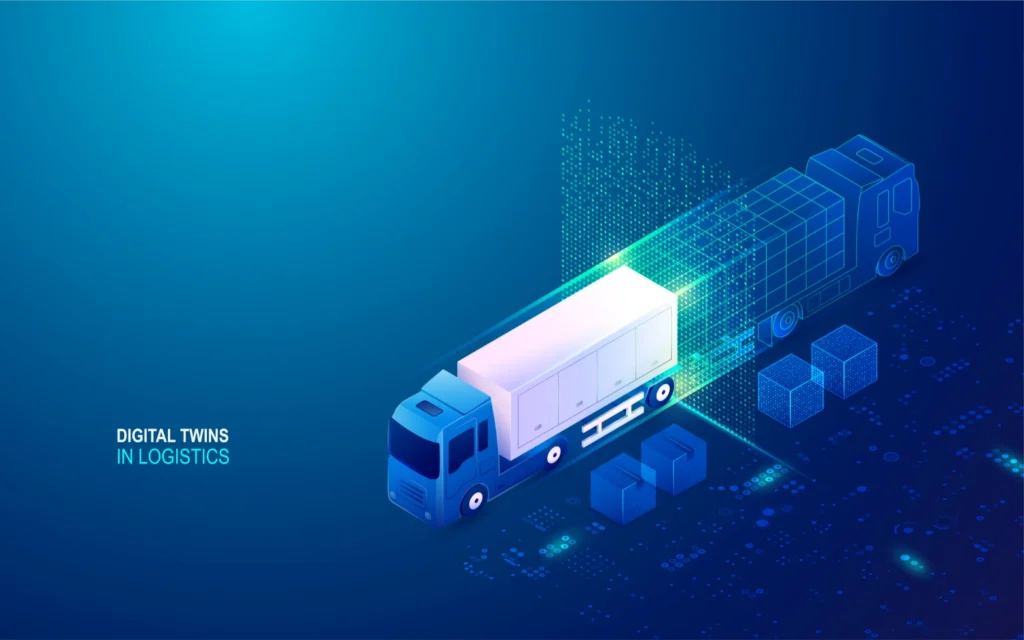
Applications of Digital Twins in Manufacturing
Digital twins can be used effectively for different activities in the entire production cycle such as product design and development, production planning, quality management, and supply chain optimization.
In product design and development, digital twins enable virtual prototyping and simulation, which is an alternative to physical prototypes, and this makes the design process faster. They are used as tools for the optimization and validation of designs through simulating various iterations, detecting possible flaws early on, and choosing the best design based on its performance, cost, and manufacturability. Therefore, a fast launch without the risk of product failure is possible thanks to the drastically cut down time-to-market and prototyping costs.
In process optimization and production planning, digital twins can simulate production processes, analyze bottlenecks and inefficiencies, and optimize production schedules, queues, and resources to achieve maximum efficiency. They allow running predictive maintenance and asset management tasks by analyzing the performance and determining when the preventive service is required. It reduces the possibility of machine failure and makes the production process more efficient.
In Quality Management Digital Twins keeps a check on the production process in real time and it picks the inconsistencies and the variances to fix the discrepancies and to maintain quality. They are helpful in diagnosing Root-Cause Analysis (RCA) and defect prevention by analyzing data from different sources ensuring it is harmonized at all production runs and facilities.
Digital Twins are beneficial to supply chain management by providing real-time visibility to fast-moving inventory that enables visibility for optimization and reduces excess or shortages. They are helpful in logistics planning and reduce costs of transport by optimizing routes. They improve the quality of delivery time as well. Digital twins in the same way enable the realization of just-in-time and just-in-sequence production strategies using resources in a more efficient manner, thus reducing inventory levels and cutting down on cash outlays.
Case Studies
Honeywell, a multinational corporation identifying itself with industrial technologies, has adopted cloud-based digital twin technology to better its manufacturing processes. Using their Connected Performance Services (CPS) platform, Honeywell integrates sensor data and devices in real-time with virtual simulation on the basis of digital twins. This integration can manage predictive maintenance, process optimization, and operational developments thereby, create significant value for their customers.
BMW, a well-known company in the automotive industry, has adopted cloud-based twin technology to streamline its production processes. Siemens’ Xcelerator portfolio with MDTE (Manufacturing Digital Twin) of Azure Digital Twins helps BMW to virtualize their production systems, perform simulations, and optimize them. The use of these digital twins at BMW has helped the company to achieve efficient operations, high-quality control, and lower production costs.
Notably, Rolls-Royce, a respected manufacturer of aerospace and marine engineering products, has implemented cloud-based digital twins that are changing the way maintenance and servicing operations are done. Their digital “twins” integrate data from engines and aircraft systems, which allows predictive maintenance and improves fuel efficiency. The systematic cost saving, the rise in safety standards, and the all-around enhancement are but a few of the rewards attained for Rolls-Royce’s digital twin technology customers.
Benefits of Digital Twins
Digital twin utilization in manufacturing processes has several benefits, such as more successful product design and testing during the R&D phase before production. Digital twins bring extra efficiency by simulating and tracking production systems thus improving performance. They help configure the product end-of-life strategies that involve proper mechanics handling of products at the end of their useful life e.g. recycling, reusing, or repurposing the materials. Furthermore, they can eliminate expensive prototyping, downtime, waste, and inefficiencies which in turn give the data-driven technologies an upper hand in the decision-making process. This way, digital twins support cost-effective production and quality assurance, increasing consumer satisfaction and brand awareness. Besides, they facilitate shorter time-to-market by speeding up product innovation and improving the efficiency of manufacturing processes.
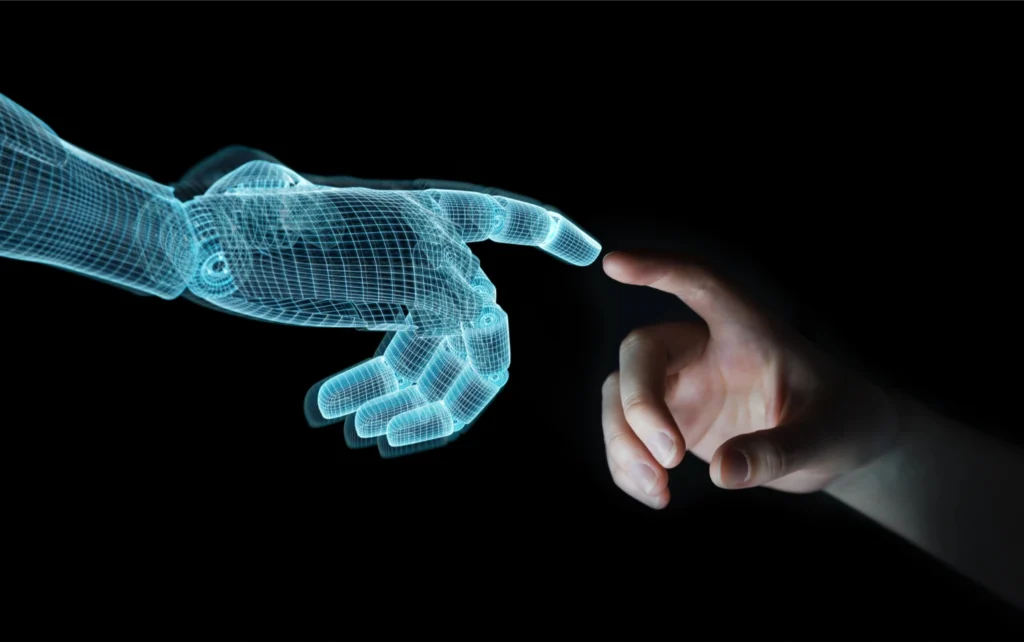
The Future of Digital Twins
Digital twins in manufacturing have a huge impact on the way manufacturing operates due to the advances in technology and the widespread use of IoT devices and data analysis. Thus, manufacturers will have the ability to cut costs, boost their competitiveness, and find an extraneous source of innovation. Possible future developments comprise the integration of generative AI and language models to improve the interface quality and autonomous decision-making capabilities for product improvement recommendations. The development of information-sharing ecosystems and platforms based on collaborative digital twin can improve the coordination of manufacturing firms, suppliers, and partners within the chain of supply providing more effective and joined activities. Furthermore, the progression in the Internet of Things technology, Artificial Intelligence, and other technologies is expected to make digital twins more accurate and capable.
Conclusion
In conclusion, digital twins are transforming manufacturing by allowing real and virtual counterparts of assets, processes, and systems to be created. Employing data integration and real-time monitoring, simulation and optimization systems, digital twins give companies a chance to improve efficiency, reduce costs, and make better data-driven decisions. With digital twins finding applications in the design of products, making plans of production, quality management, and supply chain optimization; the benefits associated with digital twins include increased productivity, reduced costs, better problem solving, better quality and customer satisfaction, and faster time-to-market. The obstacles including data integration, interoperability, cybersecurity, and workforce ready for the digital twins must be worked on while the benefits are also the aspect to be exploited to advance the digital efforts for competitive and future-proof operations. Moreover, the development of IoT will lead to future improvements in AI,5G, generative AI, digital twin, and ecosystems that will further enhance their operations and impact. The fact that early adopters are already discovering digital twins’ transformative potential may be a competitive edge that can lead to innovation and enable them to thrive in the transforming manufacturing environment.